Whether getting directions from Google Maps, personalized job recommendations from LinkedIn, or nudges from a bank for new products based on our data-rich profiles, we have grown accustomed to having artificial intelligence (AI) systems in our lives.
But are AI systems fair? The answer to this question, in short—not completely. Further complicating the matter is the fact that today’s AI systems are far from transparent.
Think about it: The uncomfortable truth is that generative AI tools like ChatGPT—based on sophisticated architectures such as deep learning or large language models—are fed vast amounts of training data which then interact in unpredictable ways. And while the principles of how these methods operate are well-understood (at least by those who created them), ChatGPT’s decisions are likened to an airplane’s black box: They are not easy to penetrate.
So, how can we determine if “black box AI” is fair? Some dedicated data scientists are working around the clock to tackle this big issue.
One of those data scientists is Gareth James, who also serves as the Dean of Goizueta Business School as his day job. In a recent paper titled “A Burden Shared is a Burden Halved: A Fairness-Adjusted Approach to Classification” Dean James—along with coauthors Bradley Rava, Wenguang Sun, and Xin Tong—have proposed a new framework to help ensure AI decision-making is as fair as possible in high-stakes decisions where certain individuals—for example, racial minority groups and other protected groups—may be more prone to AI bias, even without our realizing it.
In other words, their new approach to fairness makes adjustments that work out better when some are getting the short shrift of AI.
Unpacking Bias in High-Stakes Scenarios
Dean James and his coauthors set their sights on high-stakes decisions in their work. What counts as high stakes? Examples include hospitals’ medical diagnoses, banks’ credit-worthiness assessments, and state justice systems’ bail and sentencing decisions. On the one hand, these areas are ripe for AI-interventions, with ample data available. On the other hand, biased decision-making here has the potential to negatively impact a person’s life in a significant way.
In the case of justice systems, in the United States, there’s a data-driven, decision-support tool known as COMPAS (which stands for Correctional Offender Management Profiling for Alternative Sanctions) in active use. The idea behind COMPAS is to crunch available data (including age, sex, and criminal history) to help determine a criminal-court defendant’s likelihood of committing a crime as they await trial. Supporters of COMPAS note that statistical predictions are helping courts make better decisions about bail than humans did on their own. At the same time, detractors have argued that COMPAS is better at predicting recidivism for some racial groups than for others. And since we can’t control which group we belong to, that bias needs to be corrected. It’s high time for guardrails.
A Step Toward Fairer AI Decisions
Enter Dean James and colleagues’ algorithm. Designed to make the outputs of AI decisions fairer, even without having to know the AI model’s inner workings, they call it “fairness-adjusted selective inference” (FASI). It works to flag specific decisions that would be better handled by a human being in order to avoid systemic bias. That is to say, if the AI cannot yield an acceptably clear (1/0 or binary) answer, a human review is recommended.
To test the results for their “fairness-adjusted selective inference,” the researchers turn to both simulated and real data. For the real data, the COMPAS dataset enabled a look at predicted and actual recidivism rates for two minority groups, as seen in the chart below.
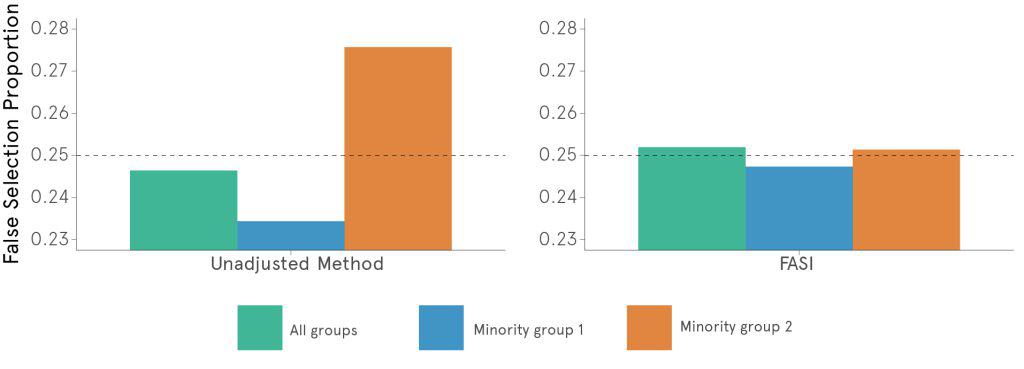
In the figures above, the researchers set an “acceptable level of mistakes” – seen as the dotted line – at 0.25 (25%). They then compared “minority group 1” and “minority group 2” results before and after applying their FASI framework. Especially if you were born into “minority group 2,” which graph seems fairer to you?
Professional ethicists will note there is a slight dip to overall accuracy, as seen in the green “all groups” category. And yet the treatment between the two groups is fairer. That is why the researchers titled their paper “a burden shared is a burdened halved.”
Practical Applications for the Greater Social Good
“To be honest, I was surprised by how well our framework worked without sacrificing much overall accuracy,” Dean James notes. By selecting cases where human beings should review a criminal history – or credit history or medical charts – AI discrimination that would have significant quality-of-life consequences can be reduced.
Reducing protected groups’ burden of bias is also a matter of following the laws. For example, in the financial industry, the United States’ Equal Credit Opportunity Act (ECOA) makes it “illegal for a company to use a biased algorithm that results in credit discrimination on the basis of race, color, religion, national origin, sex, marital status, age, or because a person receives public assistance,” as the Federal Trade Commission explains on its website. If AI-powered programs fail to correct for AI bias, the company utilizing it can run into trouble with the law. In these cases, human reviews are well worth the extra effort for all stakeholders.
The paper grew from Dean James’ ongoing work as a data scientist when time allows. “Many of us data scientists are worried about bias in AI and we’re trying to improve the output,” he notes. And as new versions of ChatGPT continue to roll out, “new guardrails are being added – some better than others.”
“I’m optimistic about AI,” Dean James says. “And one thing that makes me optimistic is the fact that AI will learn and learn – there’s no going back. In education, we think a lot about formal training and lifelong learning. But then that learning journey has to end,” Dean James notes. “With AI, it never ends.”
Goizueta faculty apply their expertise and knowledge to solving problems that society—and the world—face. Learn more about faculty research at Goizueta.